AI’s Role in Predicting Glaucoma Progression: A Game-Changer in Eye Health
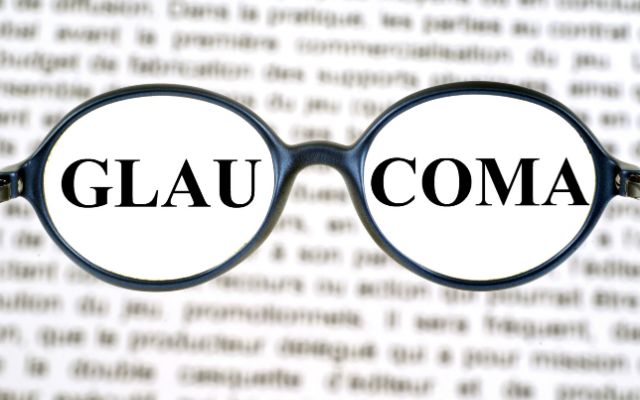
AI’s role in predicting glaucoma progression is becoming increasingly crucial in early diagnosis and personalized treatment plans. Imagine a world where artificial intelligence (AI) can predict the risk of developing glaucoma, the world’s leading cause of irreversible blindness. Researchers from Seoul National University Hospital in South Korea have been exploring this fascinating possibility, making significant strides in the field of ophthalmology. Their study focuses on training AI to recognize early warning signs in retinal images, offering hope for early diagnosis and personalized treatment plans for individuals at risk of glaucoma.
Glaucoma: A Silent Threat
Glaucoma is a formidable adversary for doctors. It stealthily progresses, often showing minimal symptoms until it’s too late. One of the primary challenges in diagnosing glaucoma is the absence of abnormally high intraocular pressure (IOP), a cardinal diagnostic feature. Without this telltale sign, predicting who will develop glaucoma and who won’t is a puzzle that researchers have long sought to solve.
While AI has made impressive strides in various medical applications, the detection of glaucoma progression remained a challenge. In this article, we’ll delve into the groundbreaking study conducted by Professor Ki Ho Park and his team, exploring the integration of AI into ophthalmology to predict glaucoma progression. Their findings, though not without limitations, offer a promising glimpse into the future of eye health.
The Promise of Deep Learning Models
Professor Ki Ho Park, the corresponding author of the study and a leading figure in the Department of Ophthalmology at Seoul National University Hospital, expresses optimism regarding the potential of AI in predicting glaucoma progression. “Our results suggest that deep learning models that have been trained on both ocular images and clinical data have the potential to predict disease progression in glaucoma suspect patients,” he says. He believes that with more extensive training and testing on a larger dataset, these deep learning models can become even more accurate.
The team’s goal is to equip clinicians with tools that can predict the individual disease courses of glaucoma suspect patients. Such personalized insights can be invaluable in tailoring management options and improving patient outcomes. But how exactly did they achieve these remarkable results?
The Study’s Methodology
To develop their predictive AI model, the research team reviewed clinical data from 12,458 eyes with suspicious early signs of glaucoma. From this extensive dataset, they honed in on 210 eyes that had progressed to full-blown glaucoma and 105 that had not. Importantly, all of these eyes were monitored every 6-12 months for a minimum of seven years.
The team’s approach integrated red flag signs observed in retinal images captured during the monitoring period with 15 key clinical features. These features included age, sex, IOP, corneal thickness, retinal nerve layer thickness, blood pressure, and body mass index (BMI). By leveraging this comprehensive dataset, they created a set of predictive combinations.
The AI Breakthrough
These predictive combinations were then fed into three machine learning classifiers, sophisticated algorithms designed to automatically order or categorize data. The outcome was nothing short of remarkable. All three algorithms demonstrated outstanding performance, consistently predicting glaucoma progression with a high degree of accuracy, ranging from 91% to 99%.
The implications of these results are profound. With the ability to predict the course of glaucoma on an individual patient basis, clinicians can offer tailored management options. This includes determining the optimal follow-up duration, deciding when to initiate IOP-lowering treatments, and establishing the target IOP levels. These personalized insights can lead to more effective and efficient patient care.
Limitations and Future Prospects
While the findings of this study are indeed promising, the research team acknowledges certain limitations. For instance, the AI model’s training data were based on a relatively small dataset, potentially limiting the model’s generalizability. Additionally, the study only included individuals with normal IOP who had not received any glaucoma treatment during the monitoring period. These restrictions may have influenced the study’s outcomes.
However, these limitations should not overshadow the groundbreaking potential of AI in predicting glaucoma progression. As technology advances and more comprehensive datasets become available, the accuracy and reliability of these predictive models are likely to improve.
Conclusion
The Seoul National University Hospital study has shed light on a remarkable breakthrough in the field of ophthalmology. By harnessing the power of AI, researchers are moving closer to predicting glaucoma progression in individuals with early signs of the disease. This progress offers hope for countless individuals at risk of losing their sight to glaucoma.
With the ability to tailor management options and treatment plans to each patient’s unique needs, AI is poised to revolutionize the way we approach eye health. As we look to the future, we can anticipate further advancements in AI-driven diagnostic tools and treatment strategies, ultimately making a significant impact on our ability to combat glaucoma and other sight-threatening conditions.
While the road ahead may present challenges and uncertainties, one thing is clear: AI has the potential to transform the landscape of eye care, bringing personalized, proactive, and effective solutions to those at risk of glaucoma. As researchers continue to push the boundaries of what AI can achieve, the future looks brighter for the preservation of sight and the prevention of needless blindness.